Algorithmic Trading
Algo Trading
Get Started - Algo Trading
Algorithmic Trading
Algo Trading
Quant
Current Trends in Algorithmic Trading

AI and Machine Learning
AI and Machine Learning enhancing the ability of algorithms to analyze vast amounts of data and adapt to changing market conditions.

Quantum Computing
Quantum computing has the potential to revolutionize algorithmic trading by processing complex calculations at unprecedented speeds.

Decentralized Finance (DeFi)
DeFi platforms are enabling new forms of algorithmic trading without traditional intermediaries.

ESG Investing
Algorithms are increasingly being used to incorporate environmental, social, and governance factors into trading strategies.
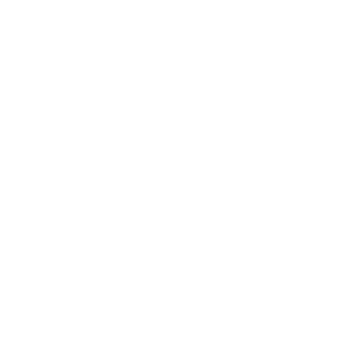
Personalization via Robo-Advisors
Algorithms will increasingly tailor investment strategies to individual investor preferences.
Tools for algo trading
Key Components of
Algo Trading
Algorithmic trading (algo trading) uses computer programs to automate trading decisions, executing orders based on predefined criteria like price, timing, or volume. By leveraging complex mathematical models, these algorithms analyze vast datasets and act faster than any human could.

Data Feeds
Access to real-time and historical market data is crucial for making informed trading decisions.

Strategy Development
A well-defined strategy is essential, including entry and exit points, risk management, and position sizing.
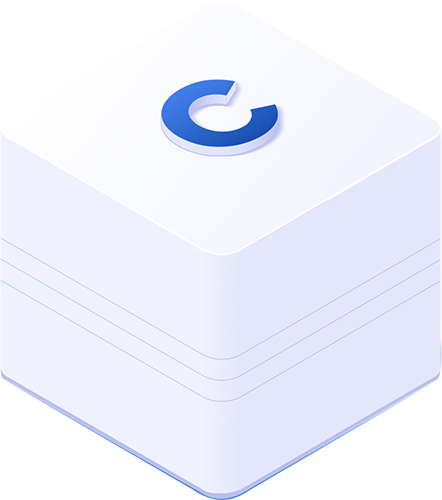
Backtesting
Traders can test their strategies against historical data to evaluate performance before deploying them in live markets.

Technology and Tools
Information on the latest tools and technologies used in algorithmic trading, including programming languages like Python and R.

Algorithms
At the heart of algorithmic trading are the algorithms themselves. These are mathematical models that analyze market data and make trading decisions based on specific rules.
Pricing
Start Something Big !
What is Algorithmic Trading?
Algorithmic trading, often referred to as algo trading, involves the use of computer algorithms to automate trading decisions. These algorithms are designed to execute trades based on predefined criteria, such as price, volume, and timing. The primary goal of algorithmic trading is to maximize profits while minimizing risks and costs.
Key Components of Algorithmic Trading
Algorithms: At the heart of algorithmic trading are the algorithms themselves. These are mathematical models that analyze market data and make trading decisions based on specific rules.
Market Data: Algorithmic trading relies heavily on real-time market data, including price quotes, volume, and historical data. This data is used to inform trading decisions and optimize strategies.
Execution Systems: Once a trading signal is generated by the algorithm, it must be executed through a trading platform. Execution systems are responsible for placing orders in the market quickly and efficiently.
Backtesting: Before deploying an algorithm in live trading, it is essential to backtest it against historical data. This process helps traders evaluate the performance of their strategies and make necessary adjustments.
Benefits of Algorithmic Trading
Algorithmic trading offers several advantages over traditional trading methods:
Speed: Algorithms can analyze vast amounts of data and execute trades in milliseconds, allowing traders to capitalize on market opportunities that may be missed by human traders.
Accuracy: Automated trading reduces the risk of human error, ensuring that trades are executed according to the predefined strategy without emotional interference.
Backtesting and Optimization: Traders can test their strategies against historical data to assess performance and make improvements before deploying them in live markets.
Diversification: Algorithmic trading allows traders to manage multiple accounts and strategies simultaneously, increasing diversification and reducing risk.
Cost Efficiency: By automating trading processes, traders can reduce transaction costs and improve overall profitability.
Challenges of Algorithmic Trading
While algorithmic trading offers numerous benefits, it also comes with its own set of challenges:
Technical Complexity: Developing and maintaining trading algorithms requires a solid understanding of programming, mathematics, and financial markets.
Market Risks: Algorithms can amplify market risks, especially during periods of high volatility. A poorly designed algorithm can lead to significant losses.
Data Quality: The effectiveness of an algorithm depends on the quality of the data used. Inaccurate or incomplete data can lead to erroneous trading decisions.
Regulatory Compliance: Algorithmic trading is subject to regulatory scrutiny. Traders must ensure that their algorithms comply with relevant regulations to avoid penalties.
Overfitting: There is a risk of overfitting algorithms to historical data, which can result in poor performance in live trading conditions.
Popular Algorithmic Trading Strategies
There are several algorithmic trading strategies that traders can employ, each with its own unique approach:
Trend Following: This strategy involves identifying and following market trends. Algorithms are designed to buy when prices are rising and sell when prices are falling.
Mean Reversion: Mean reversion strategies assume that prices will revert to their historical averages. Algorithms identify overbought or oversold conditions and execute trades accordingly.
Arbitrage: Arbitrage strategies exploit price discrepancies between different markets or instruments. Algorithms can quickly identify and capitalize on these opportunities.
Market Making: Market-making algorithms provide liquidity to the market by placing buy and sell orders. They profit from the bid-ask spread.
Statistical Arbitrage: This strategy involves using statistical models to identify mispriced assets. Algorithms execute trades based on statistical relationships between different securities.
Getting Started with Algorithmic Trading
If you’re interested in diving into the world of algorithmic trading, here are some steps to help you get started:
1. Educate Yourself
Before you begin, it’s essential to have a solid understanding of financial markets, trading concepts, and programming. Consider taking online courses, reading books, and following reputable blogs and forums.
2. Choose a Programming Language
Most algorithmic trading strategies are developed using programming languages such as Python, R, or C++. Python is particularly popular due to its simplicity and extensive libraries for data analysis and machine learning.
3. Select a Trading Platform
Choose a trading platform that supports algorithmic trading. Many brokers offer APIs (Application Programming Interfaces) that allow you to connect your algorithms to their trading systems.
4. Develop Your Algorithm
Start by developing a simple trading algorithm based on a specific strategy. Focus on defining clear entry and exit points, risk management rules, and any other parameters that will guide your trading decisions.
5. Backtest Your Strategy
Once your algorithm is developed, backtest it using historical market data. This will help you evaluate its performance and make necessary adjustments. Look for metrics such as win rate, drawdown, and overall profitability.
6. Paper Trade
Before committing real capital, consider paper trading your algorithm. This allows you to test your strategy in real-time market conditions without financial risk. Monitor its performance and make further refinements as needed.
7. Go Live
After thorough testing and refinement, you can deploy your algorithm in a live trading environment. Start with a small amount of capital to minimize risk while you gain experience.
8. Monitor and Optimize
Continuously monitor your algorithm’s performance and make adjustments as necessary. Market conditions can change, and your algorithm may need to be optimized to adapt to new trends or volatility.
Conclusion
Algorithmic trading represents a significant advancement in the way traders approach the markets. By leveraging technology and data analysis, traders can execute strategies with speed and precision. While it comes with its own set of challenges, the potential rewards make it an attractive option for both novice and experienced traders. By following the steps outlined in this guide, you can embark on your journey into the world of algorithmic trading and develop strategies that align with your trading goals.
Mobile App
BLACK BOX
WHITE BOX
Lorem ipsum dolor sit amet, consectetur adipiscing elit. Ut elit tellus, luctus nec ullamcorper mattis, pulvinar dapibus leo.Lorem ipsum dolor sit amet, consectetur adipiscing elit.
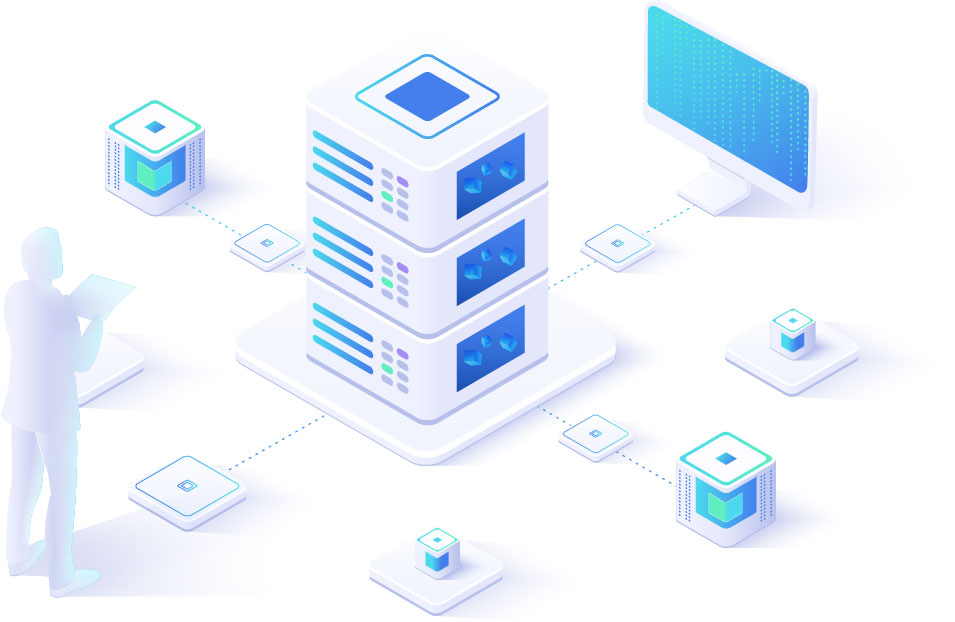
Services
AI Development: Cutting-Edge Solutions for Your Industry
Advanced Topics in Algorithmic Trading
- Machine Learning: Explore how machine learning techniques can enhance trading strategies by analyzing vast amounts of data to identify patterns and make predictions.
- High-Frequency Trading (HFT): Discuss the intricacies of HFT, where algorithms execute a large number of orders at extremely high speeds, often capitalizing on small price discrepancies.
- Sentiment Analysis: Investigate how natural language processing can be used to gauge market sentiment from news articles, social media, and other sources to inform trading decisions.
- Risk Management Techniques: Delve into advanced risk management strategies that can be integrated into algorithms to protect against significant losses.

Advanced Topics in Algorithmic Trading
Advanced Topics in Algorithmic Trading
Tools and Resources
- Backtesting Software: Recommend software tools that allow traders to backtest their algorithms against historical data to evaluate performance before live trading.
- Educational Resources: Curate a list of books, online courses, and webinars that provide in-depth knowledge about algorithmic trading and quantitative finance.
Future Trends in Algorithmic Trading
- Blockchain Technology: Discuss the potential impact of blockchain on trading, including decentralized exchanges and smart contracts.
- AI and Automation: Explore how advancements in artificial intelligence and automation are shaping the future of trading strategies and market analysis.
- Ethical Considerations: Address the ethical implications of algorithmic trading, including market manipulation and the responsibility of traders to act fairly.
Networking and Collaboration
- Industry Conferences: Highlight key conferences and events where traders can network, learn, and share insights about algorithmic trading.
- Collaborative Projects: Encourage collaboration among traders and developers to create innovative trading solutions and share knowledge.
Future Outlook for Algorithmic Trading
The future of algorithmic trading looks promising, with several predictions on the horizon:
- Advancements in AI: Continued improvements in AI will lead to more sophisticated trading strategies.
- Blockchain Integration: The use of blockchain technology may enhance transparency and security in trading.
- Personalization via Robo-Advisors: Algorithms will increasingly tailor investment strategies to individual investor preferences.
Challenges and Risks of Algorithmic Trading
While algorithmic trading has many benefits, it also comes with challenges and risks:
- Technical Issues: System failures or bugs can lead to significant losses.
- Data Quality: Poor-quality data can result in inaccurate trading signals.
- Overfitting: Models that are too complex may perform well on historical data but fail in live markets.
- Regulatory Concerns: Issues like market manipulation and spoofing can arise, leading to legal repercussions.
- Cybersecurity Risks: Algorithms are vulnerable to hacking and other cyber threats.